Online image recognition refers to the ability of computer systems to identify objects, people, places, and actions in images and videos. This technology is a subset of artificial intelligence (AI) that has gained significant traction due to its vast potential applications in various fields such as security, healthcare, retail, and social media.
At its core, online image recognition uses machine learning algorithms to process and analyse visual data, making it possible for computers to 'see' and understand content in a way that mimics human vision, albeit within certain limitations.
How It Works
- The process starts with acquiring a digital image or video frame. This image serves as the input data for the recognition system.
- The image is pre-processed to enhance its quality and simplify the data it contains. This step might involve resizing, normalisation, or colour correction to make it easier for the machine-learning model to process.
- The system then extracts features from the image. Features are specific pieces of information or characteristics of the image, such as edges, textures, or colour distributions, that are significant for recognising objects within the image.
- Using a trained machine learning model, the system classifies the extracted features into predefined categories. These models are trained on vast datasets of labelled images, learning to associate specific features with particular labels or outcomes.
- Finally, the results are post-processed to ensure accuracy and are then presented in a user-friendly manner. The system might, for example, outline identified objects in bounding boxes or provide annotations.
How Image Recognition Enhances Our Shopping Experience
1. Instant Product Discovery: By simply snapping a photo of an item or uploading an image, consumers can find identical or similar products across various retailers. This eliminates the need for cumbersome keyword searches, making discovery seamless and immediate.
2. Enhanced Engagement: Retailers leveraging visual search can engage customers more deeply, providing a novel and interactive shopping experience. It turns every moment into a potential shopping opportunity, whether a consumer is browsing social media, reading a magazine, or walking down the street.
3. Curated Shopping Experiences: By understanding individual preferences through the visual characteristics of products they interact with, retailers can offer more tailored recommendations, improving the likelihood of purchase.
4. Inspiration and Discovery: Consumers are introduced to a wider range of products than they might have found through traditional search methods, helping them discover new brands and styles that align with their tastes.
5. Price Comparison and Product Information: Shoppers can quickly compare prices, read reviews, and access detailed product information by scanning an item in a physical store, empowering them to make informed decisions.
6. Augmented Reality (AR) Integration: Combining image recognition with AR allows consumers to visualize products in their own space before making a purchase, such as seeing how a piece of furniture would look in their living room.
7. Increased Conversion Rates: By reducing the friction in the product discovery process and offering personalized recommendations, retailers can see higher engagement and conversion rates.
8. Richer Customer Insights: The data gathered from visual searches provides valuable insights into consumer preferences and behaviour, informing inventory decisions, marketing strategies, and product development.
The Technology in Action
Several major retailers and tech companies have pioneered the integration of image recognition in shopping:
- Google Lens: Allows users to search what they see, offering an easy way to discover products and where to buy them.
- Pinterest Lens: Lets users snap a photo of an object to find out where to buy it online, search for similar products, or view pinboards of related items.
- Amazon Visual Search: This enables shoppers to use images to search for products on Amazon, making the vast inventory more accessible through visual navigation.
Everyday Uses of Image Recognition Technology
Image recognition technology, a key aspect of AI, has become integral to our daily lives, enhancing convenience, safety, and efficiency quietly and effectively across multiple sectors.
1. Retail and E-Commerce: Enhances the shopping experience through visual search capabilities, allowing for instant product discovery and personalized shopping experiences. This includes price comparison, product information access, and AR integration for a more immersive interaction.
2. Healthcare: Advances diagnostics by analyzing medical imagery to detect diseases at early stages, potentially outperforming human diagnostic accuracy and improving patient outcomes through early intervention.
3. Security and Surveillance: Includes facial recognition for identifying individuals in crowded spaces and license plate recognition for vehicle identification, raising critical privacy and ethical concerns.
4. Environmental Conservation: Employs the technology for monitoring wildlife populations and tracking environmental changes, aiding conservation efforts.
5. Smartphones and Gadgets: Uses in camera applications for features like autofocus, scene recognition, and AR effects. Smart devices leverage image recognition to personalize user interactions.
Top 5 Image Recognition Tools
Below are the top 5 image recognition tools, each known for specific strengths and use cases:
1. Google Cloud Vision API
Offers powerful image analysis capabilities, including label detection, face and landmark detection, OCR(Optical Character Recognition), and explicit content detection. Ideal for a wide range of applications, from creating metadata for image catalogues to building more immersive user experiences in apps.
2. Amazon Rekognition
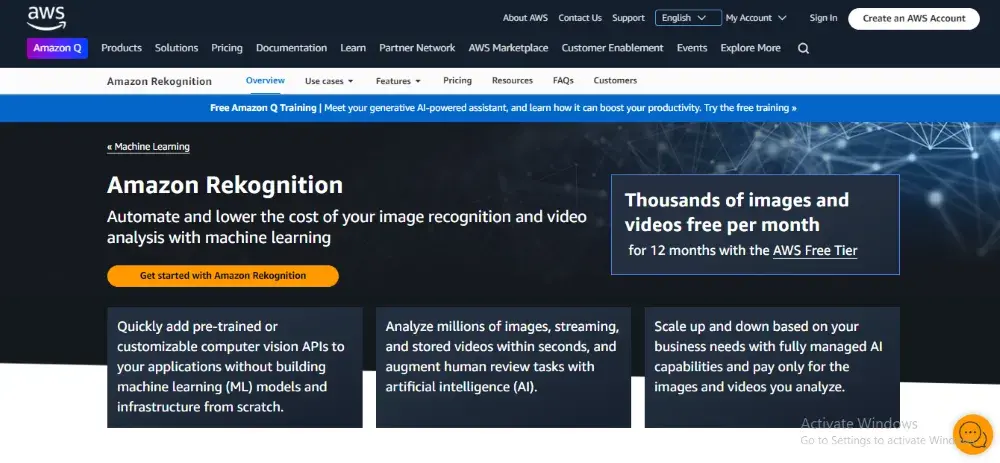
Provides highly scalable image and video analysis with facial recognition, object and scene detection, and unsafe content detection. Suitable for security and surveillance, user verification, and media content analysis, especially within the Amazon Web Services ecosystem.
3. IBM Watson Visual Recognition
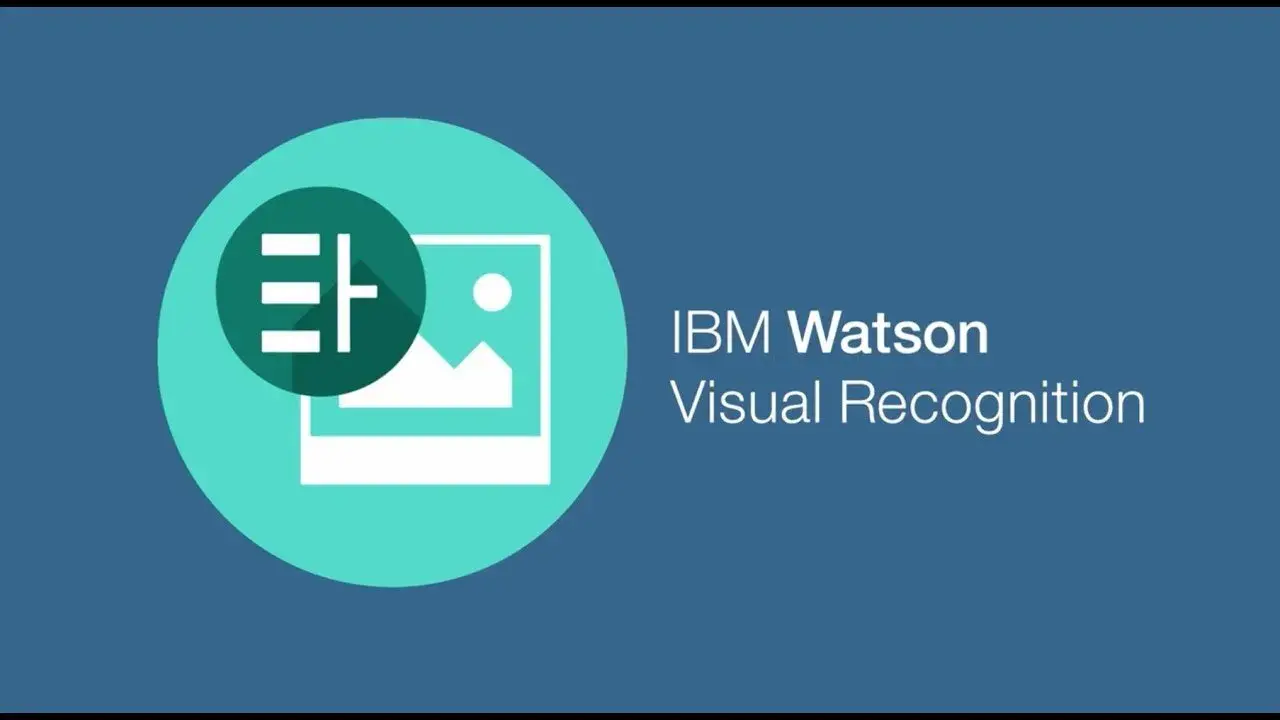
Uses machine learning to classify images, detect faces, and identify food, explicit content, and custom classes created by the user. Works well for businesses looking to automate image classification and analysis, including social media monitoring and brand management.
4. Microsoft Azure Computer Vision
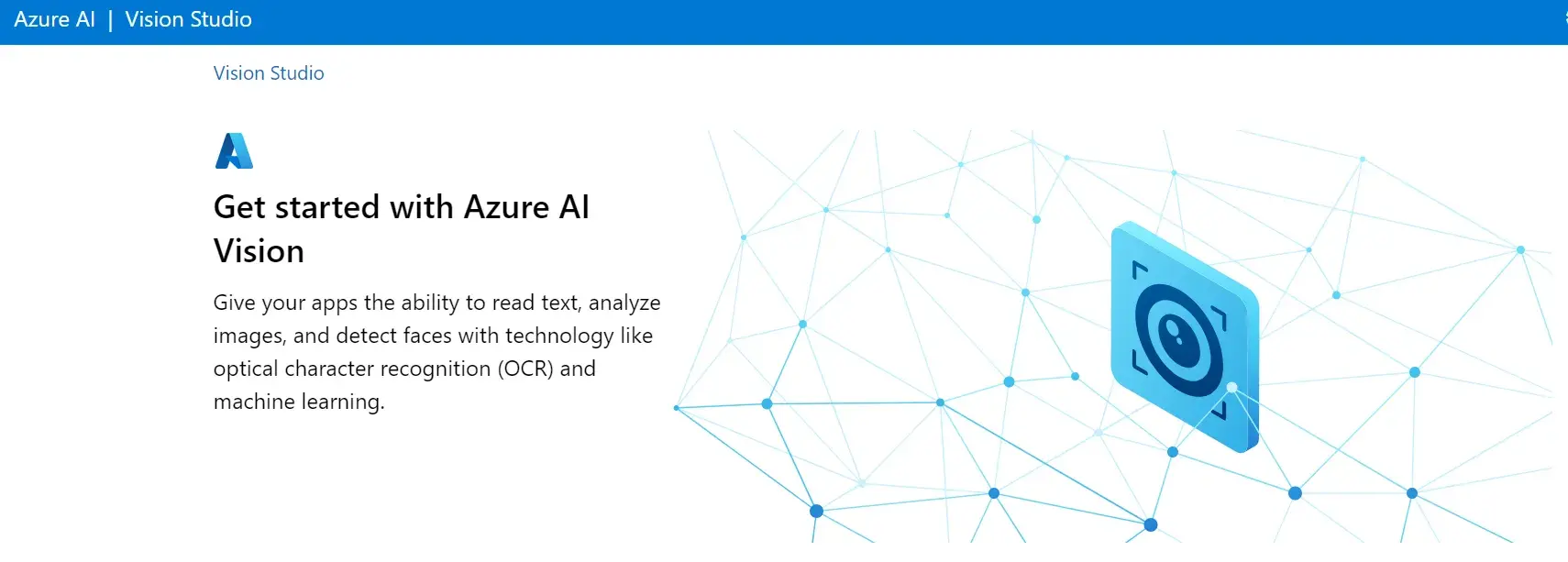
Offers capabilities like tagging visual features, detecting objects and landmarks, OCR, and generating smart image captions. Useful for developers needing to incorporate image analysis into applications or services, particularly those already using Azure services.
5. Clarifai
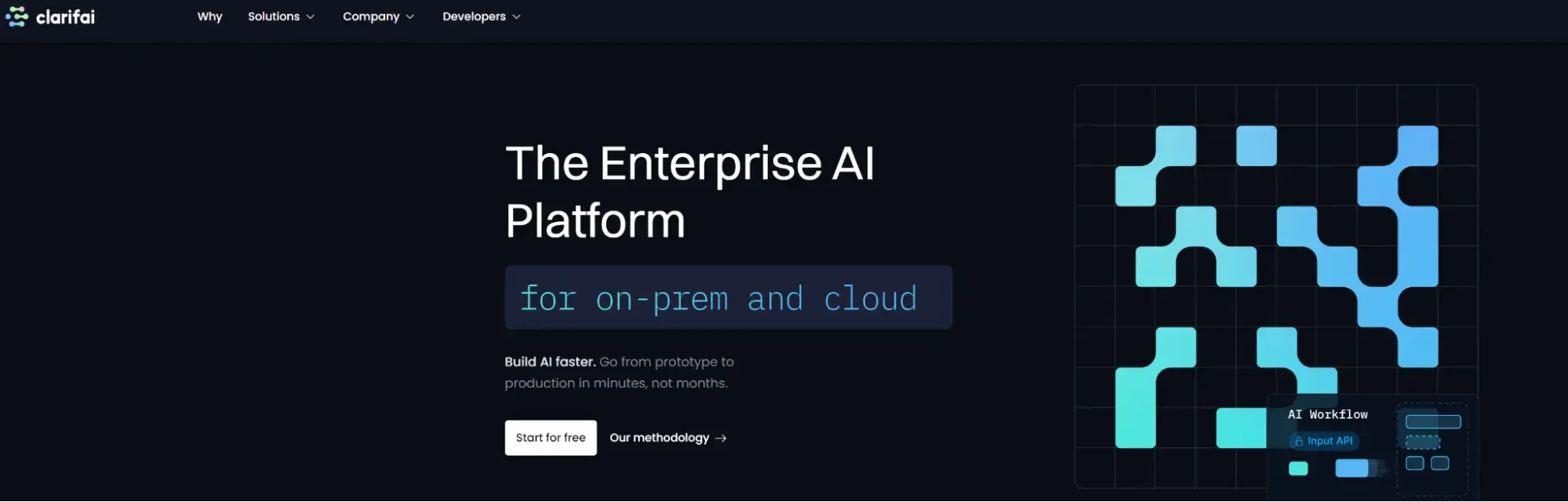
Provides advanced image and video recognition with a broad set of pre-trained models or the option to train custom models on proprietary data. Versatile for various sectors including retail, advertising, media, and security for tasks like product tagging and user-generated content moderation.
How to Choose an Image Recognition Tool
Choosing the right image recognition tool for your needs involves considering several key factors, from the nature of your application to the technical specifications and ethical considerations of the tool.
1. Define Your Requirements
Clearly define what you want to achieve with image recognition. Are you focusing on object detection, facial recognition, or pattern analysis?
Determine the scope of your project. Consider the types of images you will be processing (e.g., medical images, surveillance footage) and the environment in which the tool will be used (e.g., mobile, cloud, edge computing).
Identify key performance indicators (KPIs) for your application, such as accuracy, speed, and scalability.
2. Evaluate Technical Capabilities
Look for tools with a proven track record of high accuracy in image recognition tasks similar to your own.
Consider the processing speed of the tool, especially if real-time image analysis is crucial for your application.
Ensure the tool can be easily integrated into your existing systems and is compatible with the hardware and software environments you use.
Choose a tool that can scale with your projects, capable of processing the volume of images you expect, both now and in the future.
3. Consider the Training Data
- The tool's underlying model should be trained on high-quality, diverse datasets to ensure broad applicability and minimise bias.
- Some projects may require the tool to be fine-tuned or retrained on specific datasets. Check if the tool allows for customization and what the process entails.
4. Assess Ethical Considerations
Understand how the tool handles data privacy and security. Ensure it complies with relevant regulations (e.g., GDPR) and employs best practices for data protection.
Investigate the measures taken to mitigate bias in the tool's image recognition algorithms. Consider the tool's history and the company's commitment to ethical AI development.
5. Review Support and Community
Good technical support can be crucial, especially for complex projects. Evaluate the level of support offered by the vendor, including documentation, training, and customer service.
A strong user community can provide additional insights and resources. Check for forums, user groups, or open-source contributions related to the tool.
6. Cost Considerations
Analyze the cost structure of the tool. Consider whether it offers a one-time purchase, subscription model, or usage-based pricing.
Factor in not just the initial cost but also ongoing expenses such as updates, maintenance, and potential scaling needs.
7. Trial and Testing
Before making a final decision, take advantage of demos, free trials, or sandbox environments to test the tool's capabilities against your specific requirements.
Implement a small-scale proof of concept to validate the tool's performance and suitability for your project in a controlled environment.
Challenges and Considerations of Online Image Recognition
Challenges | Considerations |
---|---|
Data Privacy | Ensuring that the use of personal images respects user privacy and complies with global data protection regulations (e.g., GDPR). |
Bias and Fairness | Addressing biases in training datasets that can lead to inaccurate or discriminatory outcomes, especially in facial recognition. |
Accuracy and Reliability | Improving the technology to reduce errors, particularly in critical applications like security and law enforcement. |
Scalability and Performance | Ensuring the technology can efficiently process large volumes of images without compromising speed or accuracy. |
Intellectual Property Rights | Navigating the legal implications of using copyrighted images for training and recognition purposes. |
Security Concerns | Protecting image recognition systems from malicious attacks that could compromise their integrity or misuse the technology. |
Ethical Use | Considering the ethical implications of surveillance and monitoring applications, including the potential for invasive tracking without consent. |
Transparency and Explainability | Developing systems that are not only accurate but also transparent in their decision-making processes to build trust with users. |
Environmental Impact | Addressing the significant energy consumption and carbon footprint associated with training large-scale image recognition models. |
Accessibility and Inclusivity | Ensuring that online image recognition technologies are accessible to and inclusive of all users, regardless of background or ability. |
Ethical Considerations
As image recognition technology continues to evolve and integrate into various aspects of society, it brings with it a host of ethical considerations and future directions that merit careful attention.
1. Surveillance and Consent: One of the most pressing privacy concerns is the use of image recognition in surveillance systems, often without explicit consent from those being monitored. This raises questions about individuals' right to privacy and the potential for intrusive monitoring by governments, corporations, and other entities.
2. Bias and Discrimination: Image recognition algorithms can perpetuate or even exacerbate biases present in their training data, leading to unfair outcomes, particularly in facial recognition. This can affect minority groups disproportionately, calling for efforts to ensure datasets are diverse and algorithms are transparent and fair.
3. Consent and Autonomy: The use of image recognition in public and private spaces often occurs without explicit consent from those being captured by cameras. This raises questions about autonomy and the ethical use of technology in monitoring and analyzing human behaviour.
4. Data Security: The storage and processing of biometric data, such as facial recognition information, pose substantial data security risks. Breaches could lead to the misuse of personal data, including identity theft and unauthorized tracking.
The Future of Image Recognition
The future of image recognition technology is poised for transformative growth, driven by rapid advancements in artificial intelligence (AI), machine learning, and computational hardware. As these technologies continue to evolve, they are set to redefine the capabilities of image recognition in various domains, from enhancing personal devices to revolutionizing industries.
1. Enhanced Accuracy and Speed
Advancements in neural network architectures are enhancing image recognition, making models both more precise and efficient. This reduces analysis time significantly. Simultaneously, edge computing brings data processing closer to the image capture point, cutting latency and boosting speed for real-time applications in sectors like autonomous vehicles and IoT devices.
These developments are paving the way for a new era of faster, more sophisticated image recognition technologies, broadening their application across industries.
2. Improved Object Detection and Recognition
Advancements in 3D imaging are transforming system interactions with the real world, fostering innovations in AR, VR, and robotics.
Simultaneously, enhanced image recognition algorithms are improving the identification of subtly distinct objects, crucial in sectors like retail. These developments are expanding the potential and applications of image recognition technology..
3. Expansion into New Domains
Image recognition is revolutionizing healthcare diagnostics through advanced AI models that identify conditions accurately and early, enhancing patient care, while also enabling detailed monitoring of ecosystems and wildlife to support conservation efforts.
This showcases the technology's broad impact across health and environmental protection.
Case Studies
Exploring the practical applications and real-world case studies of image recognition technology reveals its transformative impact across diverse sectors.
Google AI for Diabetic Retinopathy Detection
Google developed an AI system capable of detecting diabetic retinopathy, a condition that can lead to blindness, by analyzing retinal photographs. The AI model was trained on a large dataset of retinal images, learning to identify signs of the disease with accuracy comparable to human experts.
This application of image recognition offers a scalable solution for screening diabetic retinopathy, particularly beneficial in areas with limited access to ophthalmologists, thereby preventing blindness through early detection and treatment.
New York City's Use of Facial Recognition
New York City has implemented facial recognition technology in various security applications, including identifying suspects in criminal investigations and enhancing surveillance systems. The technology compares images from crime scenes with databases of known individuals to identify matches.
While raising important discussions about privacy and ethics, facial recognition in security applications has contributed to solving crimes and enhancing public safety, showcasing the technology's potential when balanced with appropriate safeguards.
Tesla's Autopilot System
Tesla's Autopilot system uses image recognition, alongside radar and ultrasonic sensors, to enable semi-autonomous driving. The system analyzes images from cameras around the vehicle to identify road signs, lane markings, other vehicles, and obstacles.
This application demonstrates the role of image recognition in advancing autonomous driving technologies, contributing to increased safety and efficiency on the roads by supporting driver decision-making and reducing human error.
Conclusion
Image recognition technology is transforming the shopping experience by making it more personalized, efficient, and interactive. As visual search and personalized recommendations become more integrated into e-commerce platforms, the way consumers discover, interact with, and purchase products will continue to evolve.
This digital transformation not only benefits consumers by providing a more tailored shopping experience but also offers retailers innovative ways to engage with and understand their customers, ultimately driving sales and enhancing customer satisfaction.
Frequently Asked Questions
How can I use a search bar to find similar images on Google Images?
To find similar images on Google Images, go to the Google Images website, use the search bar to input your query, and press enter. You can also click on the camera icon in the search bar to upload a photo or paste the URL of an image. Google will then display images visually similar to your query or uploaded image.
What is object detection, and how does it work in computer vision?
Object detection is a computer vision technique that identifies and locates objects within an image or video. Using machine learning algorithms, the process involves analyzing the whole picture to detect and classify objects, often annotating them with bounding boxes. It is widely used in apps for various purposes, from identifying products in photos to recognizing faces.
Can I perform a reverse search with a photo from my phone?
Yes, you can perform a reverse search with a photo from your phone. Open a web browser app on your device and go to a search engine that offers image search, like Google Images. Select and drop the photo file from your phone's gallery using the upload feature. The search engine will then analyze the image and find similar photos or related information online.
What happens to my uploaded pictures when I use free image search services?
When you use free image search services to upload pictures for searching, the uploaded files are typically analyzed temporarily to find similar images or details related to the picture. Most services store the uploaded images for a short duration to process the query but do not permanently store them on their servers. Always check the website's privacy policy for specifics on how your data is handled.
How can text detection enhance the search for images online?
Text detection is a feature of computer vision that identifies and reads text within images, which can significantly enhance image search capabilities. When you search for images using keywords or upload a picture containing words, text detection algorithms analyze the content to improve the accuracy of search results. This allows search engines to return more relevant images or instances where the detected text appears, offering the best solution for finding specific information or related visuals online.
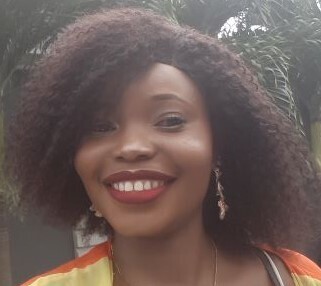
Yetunde Salami is a seasoned technical writer with expertise in the hosting industry. With 8 years of experience in the field, she has a deep understanding of complex technical concepts and the ability to communicate them clearly and concisely to a wide range of audiences. At Verpex Hosting, she is responsible for writing blog posts, knowledgebase articles, and other resources that help customers understand and use the company's products and services. When she is not writing, Yetunde is an avid reader of romance novels and enjoys fine dining.
View all posts by Yetunde Salami